What Is UEBA and Why It Should Be an Essential Part of Your Incident Response
In the world of cybersecurity, security teams are trending away from using prevention-only approaches, according a 2018 Gartner report called Market Guide for User and Entity Behavior Analytics. As security teams shift toward balancing cyber threat prevention with the newer detection and incident response (IR) approaches, they are increasingly adding technologies like user and entity behavior analytics (UEBA) to their conventional SIEMs and other legacy prevention systems in an effort to boost the effectiveness of their security systems.
The evolution of UEBA
For a basic definition, UEBA baselines user behavior and entity behavior activities, and combines it with peer group analysis, and then searches for and analyzes anomalous activity in order to detect potential or actual intrusions and malicious activity. UEBA goes beyond fact-based security and simple correlation rules, and leverages both user behavior and entity behavior-based analytics, and models threats based on individual user behaviors.
Gartner created the UEBA acronym several years ago when it renamed “user behavior” analytics (UBA). They added the “E” to emphasize the importance of “entity behavior” other than just user behavior, such as with cloud applications or unmanaged endpoints. The E “recognizes the fact that other entities besides users are often profiled in order to more accurately pinpoint threats, in part by correlating the behavior of these other entities with user behavior,” according to Gartner.
Why UEBA improves UBA and legacy SIEMs
UEBA represents an important improvement over UBA and legacy SIEM systems for a number of reasons. First, it overcomes the limitations of SIEM correlation rules—and the reality that in many cases the whole model of correlation rules is broken. Some of the problems associated with relying on SIEM correlation rules include:
- You can’t find attacks because the rules lack context, or miss incidents that have never been seen before, thus generating false negatives.
- Rules require too much maintenance.
- Improperly filtered rules can make incident response execution slow because administrators need to filter the application of rules to determine which data is relevant, and which data is irrelevant in your event landscape.
UEBA also reduces false positives, helping to eliminate alert fatigue. And by enabling teams to prioritize their alerts, UEBA makes it possible for your security experts to focus on the most credible, high-risk alerts.
Why UEBA should be part of any organization’s security framework
We all know that cyberattacks are becoming more complex and harder to find. So trying to write correlation rules for thousands of different possible scenarios is no longer practical. This is especially true in the case of insider threats. If you establish rules such as “Send an alert whenever a user sends an email attachment larger than 4MB,” you would need to consider every individual user, and then establish exceptions. For instance, graphic designers in the marketing department might routinely send large PDF files. Rather than asking your security experts to manually whitelist every case like this, UEBA replaces traditional Boolean alerts with probabilistic models or risk factors based on advanced analytics.
In this way, UEBA provides superior insider threat detection compared with conventional SIEM correlation rules. Plus, UEBA tracks anomalous user behavior and suspicious lateral movements not only within your organization/network, but it can also be associated with your cloud services, machines, mobile devices, and IoT assets. User behavior analytics can also provide dramatic time savings because teams don’t have to dig into logs in diverse locations to put together a story of the incident. A sophisticated UEBA system ingests data from all the different log sources—such as Windows AD, VPN, database, badge, file, proxy, and endpoints—and builds a contextual story around the incident for your security teams to analyze.
Here are some of the many benefits of UEBA:
- Fuses various types of risk information to make up a final score for risk ranking
- Enables prioritization and effective response
- Provides automated incident response, allowing teams to respond to security incidents rapidly and with less effort.
Models and math replace true/false alerts
Models can offer more advantages to teams using UEBA. Models that emit probabilities are more effective than true/false alerts and allow the use of data science to combine multiple pieces of evidence across different data sets to define the likelihood of a user account having been compromised or engaged in illicit activities.
With an advanced UEBA, you can model:
- The normal processes that invoke authentications, so you can catch the anomalous ones
- The time of day and the day of the week a user performs account creation, and whether it’s normal for this user to create accounts compared to their peers
- Which ports and IP addresses specific devices connect to on a regular basis
- Which users perform which power shell operations
- Subnets per user that talk to the Internet
- Which users traverse which subnets from specific critical assets
Beyond static threshold-based risk assessment
Awareness is growing that static threshold-based risk assessment no longer stands up against today’s sophisticated bad actors and tech-savvy malicious insiders.
With UEBA you can match sophisticated threats with sophisticated detection, such as risk score adjustment based on peer group-based indicators. For example, UEBA can look at anomalous behavior of one individual accountant in comparison to their peer group of accountants. Using models and matching, UEBA considers not one but multiple anomalous behaviors that vary from the peer group to indicate a threat, such as a specific accountant uncharacteristically hitting a subnet like HR.
Or, consider the case of a user coming in over VPN from Barbados for the first time. Just because the entity behavior is anomalous doesn’t mean it’s bad; the user may simply be traveling. However, UEBA would perform user analytics on related behavior such as this same user moving across multiple subnets, which would dramatically raise the risk score.
In the case of an APT, a hacker using compromised credentials might look like a run-of-the-mill employee in human resources to a legacy system. However, if the same user suddenly accesses the industrial control subnet, UEBA would recognize that user behavior as anomalous.
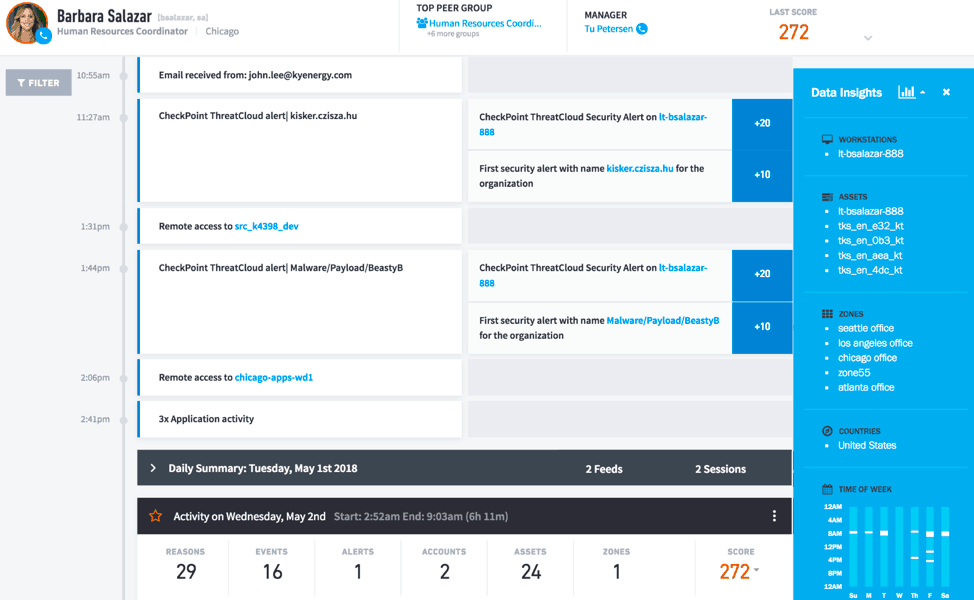
Finally, in all of these scenarios, machine learning in UEBA systems like Exabeam can help to better estimate a potential alert’s context so that you can calibrate its risk score, thereby avoiding high rates of false positives.
Learn more about User and Entity Behavior Analytics
Have a look at these articles:
- What UEBA Stands For (And a 5-Minute UEBA Primer)
- What Is UEBA and Why It Should Be an Essential Part of Your Incident Response
- Threat Detection and Response: How to Stay Ahead of Advanced Threats
- User Behavior Analytics (UBA/UEBA): The Key to Uncovering Insider and Unknown Security Threats
- Behavioral Profiling: The Foundation of Modern Security Analytics
Learn more about the Exabeam behavioral analytics capabilities.